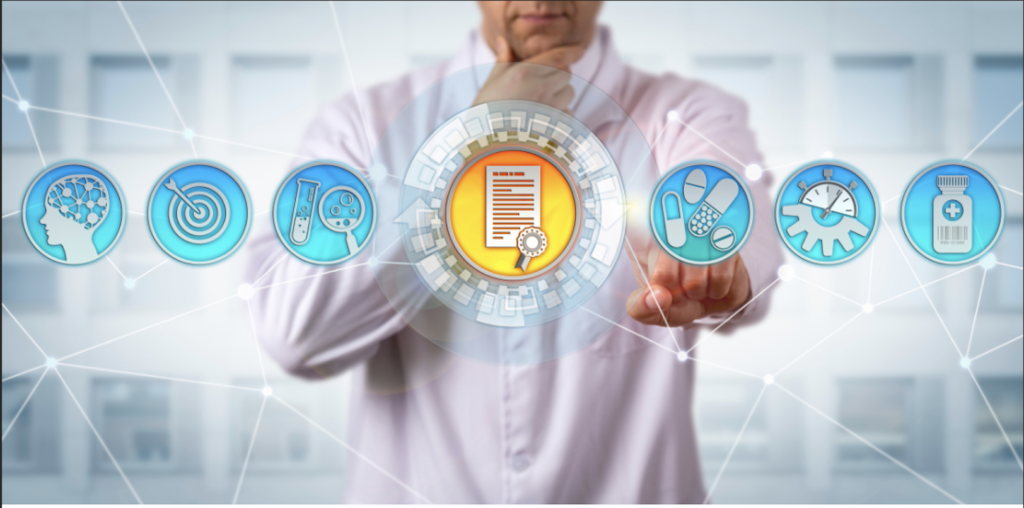
It seems as if nobody talks about anything except AI these days, especially in the drug discovery part of our industry. And why not? In a relatively short period of time, artificial intelligence and machine learning (both are terms which we dislike, by the way), are already demonstrating their collective value in discovering new targets and new candidates, several of which are already well into clinical trials in oncology, fibrosis, and other areas.
But exactly what is AI in drug discovery? If you are reading this, you probably do not need this term defined. Regardless, let us agree for the moment that AI in Drug Discovery involves the use of algorithms, data analytics, and other computational tools to analyze vast amounts of data. The resulting outputs can be used to identify potential drug targets, hits and leads, and so forth. AI is essentially a suite of tools and techniques which, when combined, are capable of processing large amounts of data, identifying patterns, and making predictions, which can help accelerate the drug discovery process and reduce the time and cost required to develop new drugs. This approach can also help repurpose existing drugs for completely new, previously unexpected indications. Thus, the key points in our view are a) the ability to analyze massive amounts of disparate data and b) the ability to propose (in silico) lead compounds which are more specific, more potent, more selective, and safer.
AI can also be used to design clinical trials, and they come with their own set of problems. For example, clinical trial data may not include data from certain patient subgroups, which can limit the effectiveness of AI algorithms in predicting drug efficacy and toxicity. However, we will not discuss clinical trials in this post.
There is ample literature and case studies describing the use of artificial intelligence in drug discovery. In one example, Molyneaux et al (Link to PDF) describe how they screened a library of (sic.) several million compounds to identify 76 candidates for further in vitro analysis. Chen, et al (Link to PDF) is a very good primer for understanding what artificial intelligence for drug discovery actually is, including data sources, techniques, and applications. Gupta, et al have a nice graphical summary of all of the potential inputs associated with artificial intelligence in drug discovery (Link to PDF), as does Paul, et al (Link to PDF). Santa Maria, et al (Link to PDF) raise some very interesting points on the non-technical challenges associated with AI, including cultural fit, communication, hype, and a competitive job market.
However, the use of AI in drug discovery is not without challenges, beginning with the quality and quantity of the training data used to build the corresponding models. AI requires large amounts of high-quality data to make accurate predictions. In many cases, the quality and quantity of available data may be limited, especially for rare diseases or conditions. In addition, the data may be biased, incomplete, or inconsistent, which can lead to inaccurate predictions. Another challenge is that AI algorithms can be prone to bias, particularly if they are trained on biased datasets. We will return to the training data issue later.
Another challenge is that AI algorithms can be prone to bias, particularly if they are trained on biased datasets. For example, if a dataset used to train an AI algorithm is biased towards a particular patient subgroup or a particular animal model, the algorithm may not be effective in predicting drug efficacy or toxicity for other subgroups.
Third, AI results can be difficult to interpret. Unlike traditional statistical models, AI algorithms do not always provide a clear explanation of how they arrived at a particular prediction or recommendation. This can be a challenge in drug discovery, as it can be difficult to identify the underlying biological mechanisms that are responsible for a particular prediction or recommendation. This can make it difficult to validate and replicate the results of AI algorithms.
And, finally, each AI system may differ from one to another, even when tasked with the exact same analysis. In other words, if we ask three AI service providers to conduct an analysis and provide a deliverable, such as a list of compounds for synthesis and testing against a particular target, it is quite likely that all three lists will be different; substantially so in some cases. This may require a completely different (and independent) analysis for choosing which compounds to synthesize and test.
~~~~~
We have been privileged to meet with a number of AI drug discovery companies over the past 12-18 months. Stepping back and reviewing their decks and web sites, a few patterns emerge.
Business Models
We see a continuum of business models here. On the one extreme, there is a Service Business Model. AI companies in this category are purely dedicated to providing their customers with a service(s), which may include activities such as novel lead generation, lead optimization, ADMET predictions, and combinations thereof. Their offerings are straightforward, in the sense that they leverage their expertise and technologies on behalf of their clients for specific projects. Once the project is completed, the relationship ends. There are no expectations of sharing in the resulting intellectual property related to the output (i.e., novel compounds identified). Any improvements in the underlying AI technology remain with the AI company. Everything is clean, simple, and straightforward. The modern CRO industry emerged in the 1980s as Big Pharma companies began to identify ways to outsource work, both to reduce costs and to leverage external expertise and capabilities. Outsourcing AI is no different, in the sense that the skills and expertise present in an AI service provider can be brought to bear on a discovery and development project for a variable period of time and cost.
On the other extreme is the Innovation Business Model. On this end of the spectrum, companies are building AI models to discover novel candidates, which will then be synthesized and tested. In many cases, these companies are building models for specific families of drug targets (such as kinases) or specific classes of candidates (such as psychedelics). These testing data can be used to further train the models, theoretically resulting in greater chances of finding candidates which are more potent and selective for the underlying target(s). Companies like this also have a standard business model, in the sense that their “pitch” is not based on the AI capabilities, but on the commercial potential of the candidates they are creating. Again, everything here is fairly simple, clean, and straightforward (relatively speaking, of course).
So what’s the problem?
Muddled Strategy – In many of the AI presentations and web sites, we see clear evidence of a very muddled strategy. Typically, these will be companies who want to be both BioTech and DeepTech (whatever that means). They want to appeal to both sets of investors. They want to develop new drugs, but they want service contracts and the corresponding revenues to extend their runways (not to mention their need for training data). This oftentimes results in tremendous amounts of conflict between management, their investors, and clients, each with different views on how the company should move forward.
Lack of Differentiation – We frequently see AI presentations and websites with very similar messages, such as:
AI can accelerate drug discovery and development
AI can reduce drug discovery and development costs
AI can help create novel drug candidates from chemical white space
AI can help discover novel candidates which are safer, more potent, and more effective
It is our belief that these statements are factually correct, as there is ample evidence that some (but not all) companies are achieving these endpoints (or at least claiming to do so). But when multiple presentations have the exact same messages, how does one AI company differentiate itself from another? And therein lies the problem.
Multiple Simultaneous Audiences – We mentioned previously that many AI companies try to appeal to two different sets of investors: the traditional biotech investors AND the “DeepTech” investors. This is two different sets of audiences, with different criteria, different pain points, and should be approached with different messages and presentations. More importantly, the Tech Bros investing in AI are unlikely to have a deep understanding of the traditional biotech business model, where returns are generated through licensing, M&A, and IPOs…all of which can occur long before a candidate makes it through approval and commercialization.
Customers as Competitors – Service providers are not only competing against each other, they are also competing against the internal capabilities and resources of their own prospective customers. This is especially the case with large, multinational companies who have their own internal AI drug discovery / computational chemistry groups. AI companies (both Service and Innovative) must quickly demonstrate they can perform an activity and/or create a candidate that their customer’s internal teams cannot. This is an extraordinarily difficult thing to do as internal customer activities are kept tightly under wraps and rarely disclosed in detail.
Back to Basics
A company can outperform rivals only if it can establish a difference that it can preserve. M Porter, 1996.
In the classic article What Is Strategy? (HBR, November-December, 1996), Michael Porter describes the fundamental characteristics of a strategy as a series of choices…choices which must balance strategic positioning and technical competitive advantage with operational effectiveness (which is not a competitive advantage in the long run for most companies in most industries). This was later followed by Collins and Rukstad piece on the importance of clearly communicating a strategy (HBR, April, 2008).
Companies leveraging AI in drug discovery are no different. On the one hand, there is a need to have internal technologies and technical capabilities which can deliver results that are unique relative to competitors. Examples may include the generation of structures of novel (and clearly patentable) candidates, the identification of novel, druggable pockets on a given target, and so on. This is obviously difficult to achieve without significant investment and development in the underlying technologies and expertise. But this needs to be married with a certain level of operational effectiveness in order to keep costs down and remain price competitive. As in other markets, there will be very few companies who have such a unique service offering that they can enjoy premium pricing and/or suboptimal operational excellence.
So, what are the solutions to these challenges?
Sell Books and Only Books –Approaching multiple (different) audiences with the same messages (whether fundraising or seeking clients) is symptomatic of a company that cannot decide what they want to be when they grow up. Focusing on a particular segment or service or niche is best, especially for young companies. Think of it this way…Amazon started off by selling books online. That’s it. Books. The next major category was…music stored on compact disks (ask your parents if you do not know what a “CD” is). AI service providers who focus on one specific strategy as a foundation for growth will be better off in the long run.
Make a Decision – Related to bookselling is the need to make decisions. In a challenging financing environment, we get the sense that companies will say just about anything to receive investment. Similarly, AI companies pursuing a service business model will make all sorts of “we can do anything” claims to generate business. This confuses the messaging, which in turn makes it more challenging to generate interest from investors and/or clients alike.
Audiences – As we have said many many many times, one “pitch deck” is never enough. Different kinds of presentation and marketing materials are needed, depending on the audience. An investor deck emailed to less sophisticated Preseed investors simply must be different than a deck presented to sophisticated Series A VCs during a 10-minute pitch event. The client acquisition plan (which may include presentations, brochures, videos, digital marketing campaign, etc.) should not include either of the aforementioned decks.
Ultimately, these solutions (and more) are variations of the same (somewhat basic) fundamental strategic concepts described by Porter and others:
> A brutally honest assessment of your positioning relative to competitors, with a clearly-defined (and clearly explainable) basis for that positioning;
> A staged business growth model which builds from strength to strength;
> A quantitative approach to implement and monitor the growth plans, with milestones and risk mitigation strategies.
P.S. Can you pick out which sentences in this post were written by ChatGPT?