At Lacerta Bio, we’ve been reading the fascinating book, The Signal and The
Noise by Nate Silver. The book is a terrific review of how and why forecasts often fail, and why some of them occasionally do succeed.
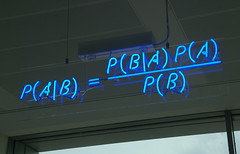
The key philosophy in the text is Silver’s avocation for a Bayesian mindset to statistics and forecasts, and the rejection of population sampling as a means to make decisions.
For us in pharma, we’re constantly exposed to population sampling in clinical trials, but is this the best way to make decisions?
Probably not.
A Bayesian approach closely mimics how a physician thinks when choosing a therapy. Before deciding on a therapy, a physician intuitively tries to imagine what might happen to that individual patient if a given therapy is chosen. Will this person be cured? Will there be a side effect? If so, which one? After all, as was noted in a 2005 article, clinicians are natural bayesians:
Two main approaches are used to draw statistical inferences: frequentist and bayesian. Both are valid, although they differ methodologically and perhaps philosophically. However, the frequentist approach dominates the medical literature and is increasingly applied in clinical settings. This is ironic given that clinicians apply bayesian reasoning in framing and revising differential diagnoses without necessarily undergoing, or requiring, any formal training in bayesian statistics. To justify this assertion, this article will explain how bayesian reasoning is a natural part of clinical decision making, particularly as it pertains to the clinical history and physical examination, and how bayesian approaches are a powerful and intuitive approach to the differential diagnosis.
In other words, a broad population/sampling data set is not ignored. But it forms the starting point for making a patient-specific decision.
We already have Bayesian models in pharmacokinetics. So why not incorporate Bayesian thinking into clinical trials?
We think it’s basically because we like to have a single number to work with. Like real options in finance, Bayesian thinking is simply not what we’re all used to. We’re not accustomed to thinking about probabilities. Just like we want a single number in finance (an NPV, a PYS, an EPS, etc.), we also want a single number in clinical development (basically, the p value).
However, it should be feasible to develop Bayesian approaches to clinical trials, and then make the resulting models available for physicians to use on the fly on their smartphones or tablets. So while there are plenty of texts and articles exploring the subject, it is time to make this more approachable and practical for clinical researchers and prescribers.
But why is this important?
Because we continue to see too many drugs fail not because of safety, but because of efficacy. Remember, a lack of efficacy in a given population does not mean that a drug will not work in an individual patient, even when that individual patient matches the study population exactly.
This is exactly where a Bayesian approach could help. That is, if we had population bases safety and efficacy data, then some candidates (not all) might have enough of an efficacy signal to warrant a “Bayesian approval.” Linking individual patient markers, diagnostic data, or other data to a drug’s population-based parameters via a Bayesian model could help physicians make more individualized treatment decisions.
Importantly, this could increase the number of drugs a prescriber has to work with, especially in areas where approvals are currently short of unmet need, e.g., cancer, CNS, and many other areas where candidates which failed their population-based trials might still have some value for individual patients.
Related articles
Book Review: The Signal and the Noise: Why So Many Predictions Fail- But Some Don’t
Bayesian vs Frequentist Statistics
Zero Dark Thirty and Bayes’ theorem
